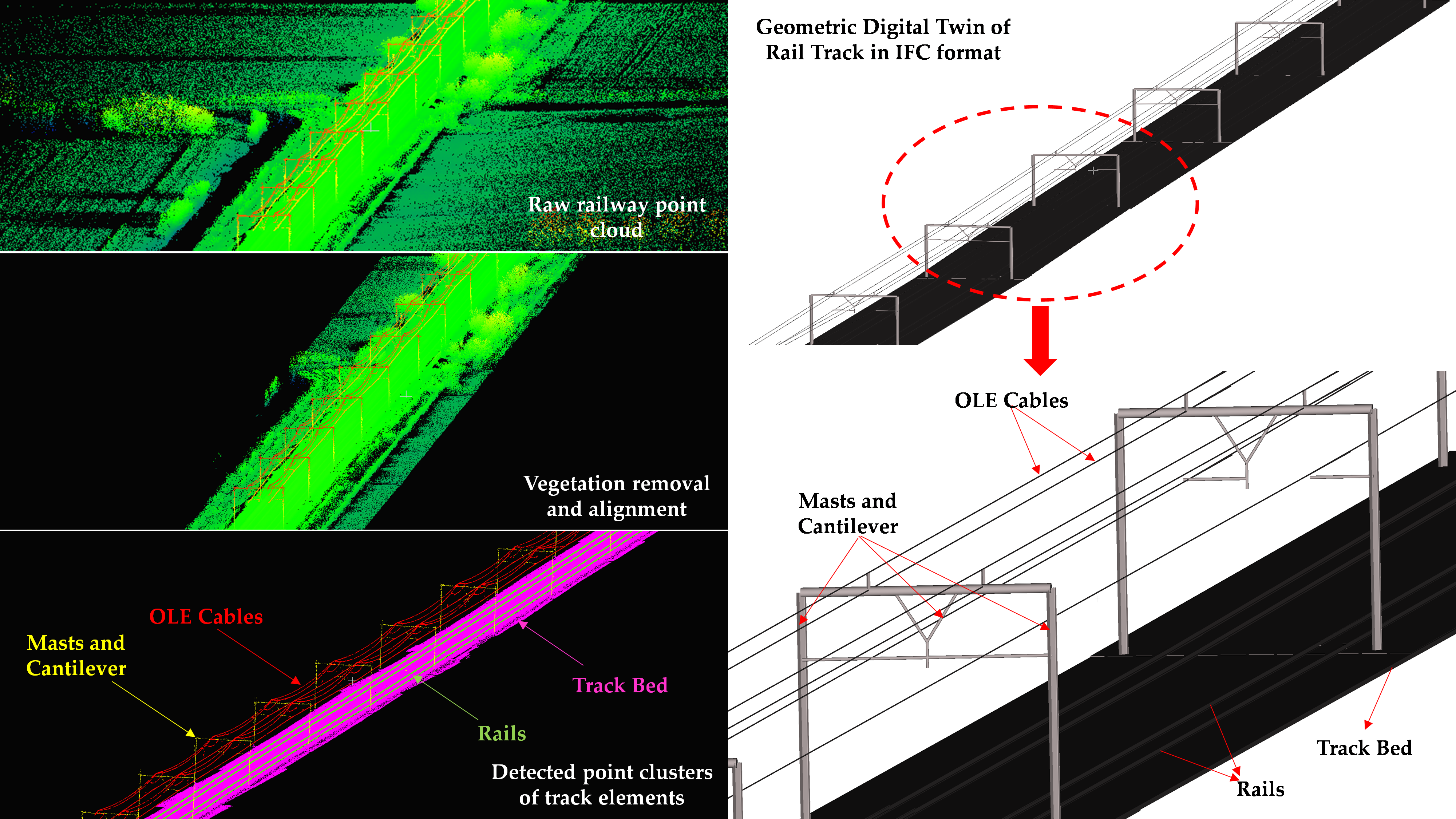
The generation of geometric railway information model, i.e. a holistic data model which has recently become known as a "Digital Twin", of an existing railway from point cloud currently counteracts the expected benefits of the digital model. This digitisation of the geometry of assets is highly reliant on manual inputs for railways, needing ten times more labour hours than scanning the physical asset. It is expected that the automation of the twinning process will reduce the modelling time and ultimately save costs. PhD Candidate Mahendrini Ariyachandra is researching methods to achieve such automation when generating the geometry-only digital twins of rail infrastructure.
The proposed method starts with tools for cleaning the point cloud data and roughly detecting its positioning and orientation. The resulting data sets are processed by restricting the search region of the masts considering its positions compared with the track centerline. Detected point clusters of masts are then used to restrict the search region of Overhead Line Equipment (OLE) that includes contact cable, catenary cable, auxiliary cable, cantilever, connecting beam and track structure elements including rails and trackbed. Resulting point clusters of the OLE elements are converged with various parametric models of different catenary configurations to verify the presence of OLE elements and to find the best possible fit while track structure elements are reconstructed using model fitting techniques. The proposed method finally outputs a geometry-only digital twin of the OLE system in Industry Foundation Classes (IFC) format. The method was tested on an 18 km railway point cloud and achieves overall detection rates over 90% F1 scores for all railway elements. The accuracy of the generated model is evaluated using distance-based metrics between the ground truth model and the automated model. The average modelling distance is less than 5 cm Root Mean Square Error (RMSE) for all 18 km data.
The originality of this method stems from its direct extractions of railway track elements by limiting their search region using the railway topology, without analysing any additional information such as scanning geometry and intensity of the input data. This is the first method that exploits railway topology knowledge as a guide to automatically extract points corresponding to railway track elements in large-scale projects and is the foundation for efficient railway track modelling from point cloud data.
For further information, contact Mahendrini Ariyachandra at mfa47@cam.ac.uk.