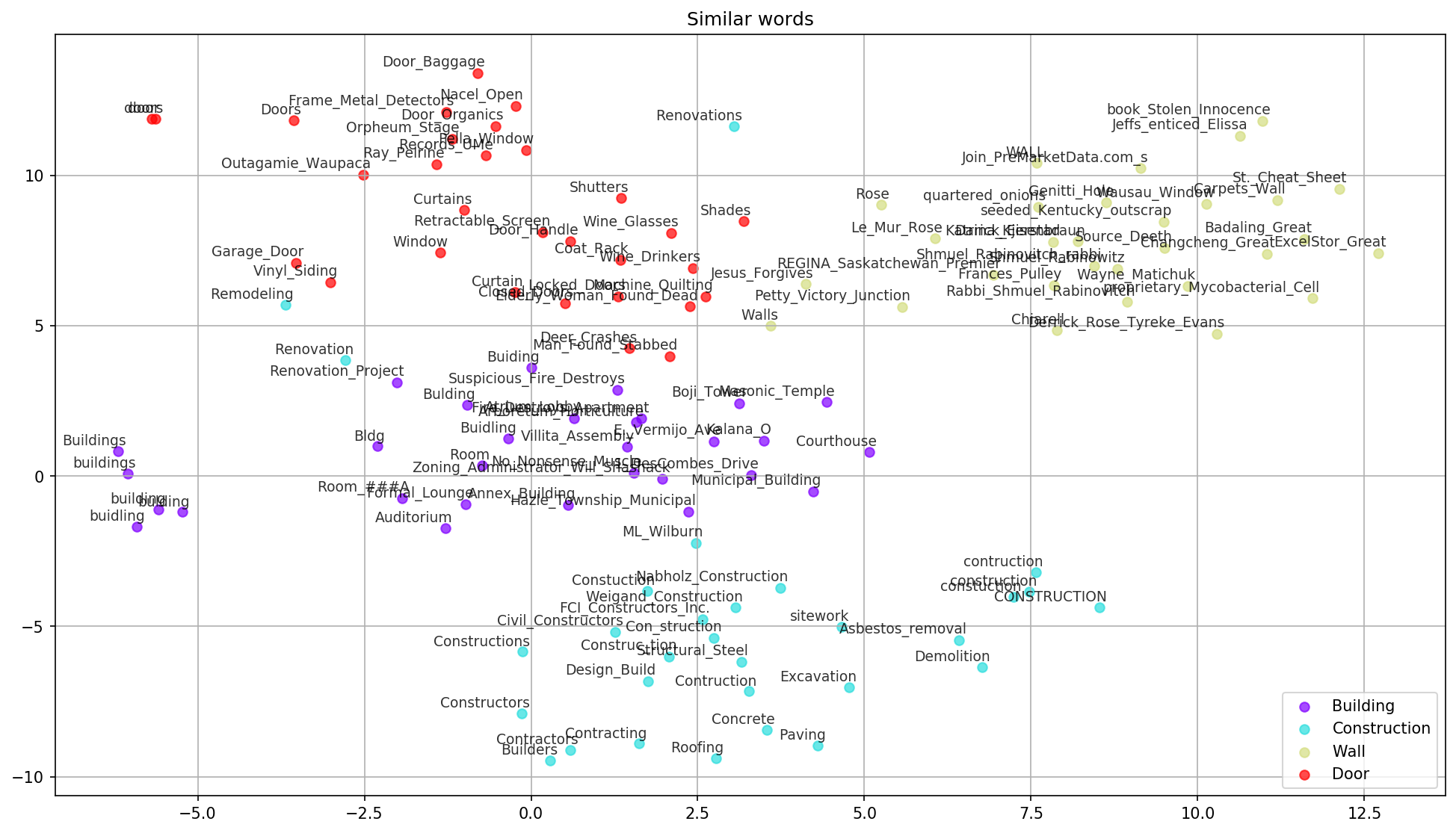
“Efficiency is the intelligent laziness.”
Efficiency is a magic word in the construction industry. Sydney Opera House, a world iconic landmark, often appears as a failure case study in construction management. This project is over budget for more than 14 times and delayed for 10 years. This problem is not unique. Crossrail showed its potential to be the twin case in the UK.
Superficially, the construction industry seems healthy and well operated. The global market is worth $10trn, and the industry employs about 7% of the world’s working-age population[1]. Improving the industry’s efficiency seems to be a key to our quality life.
With enormous growth in data, people now can leverage the data value to improve efficiency. The Cambridge team along with Kier and nPlan are approaching this problem through developing a schedule-learning platform that learned from thousands of historic projects to offer a unique and scalable solution for reliability and confidence in project planning.
During the journey, we came to understand the top three challenges that hinder the effective learning of construction schedules are poor readability, poor coherence, and lack of re-use. Human beings can learn from failure because our brain can analyse decisions made, store knowledge learned, and apply the knowledge into future similar scenarios. When processing other people’s knowledge, understanding is the key obstacle.
A construction schedule is usually created by schedulers manually in the current practice. Schedulers and project managers create, modify, and execute construction activities based on their historic experience and knowledge. However, it is challenging for people outside of the project context to understand scheduled activities and apply previous knowledge into new projects.
Any enquiries about this project, please contact Ying Hong (yh448@cam.ac.uk)
[1] McKinsey Quarterly, (2016), Beating the low-productivity trap: How to transform construction operations